目錄
- 1 下載和解壓數(shù)據(jù)集
- 2 加載庫(kù)函數(shù)
- 3 特征提取以及數(shù)據(jù)集的建立
- 建立類別標(biāo)簽字典
- 提取梅爾頻譜特征
- 獲取特征和標(biāo)簽
- 獨(dú)熱編碼
- 把數(shù)據(jù)集劃分為訓(xùn)練集和測(cè)試集
- 4 建立模型
- 搭建CNN網(wǎng)絡(luò)
- 訓(xùn)練模型
- 5 預(yù)測(cè)測(cè)試集
- 6 結(jié)果
MFCC
梅爾倒譜系數(shù)(Mel-scaleFrequency Cepstral Coefficients,簡(jiǎn)稱MFCC)。
MFCC通常有以下之過(guò)程:
- 將一段語(yǔ)音信號(hào)分解為多個(gè)訊框。
- 將語(yǔ)音信號(hào)預(yù)強(qiáng)化,通過(guò)一個(gè)高通濾波器。
- 進(jìn)行傅立葉變換,將信號(hào)變換至頻域。
- 將每個(gè)訊框獲得的頻譜通過(guò)梅爾濾波器(三角重疊窗口),得到梅爾刻度。
- 在每個(gè)梅爾刻度上提取對(duì)數(shù)能量。
- 對(duì)上面獲得的結(jié)果進(jìn)行離散傅里葉反變換,變換到倒頻譜域。
- MFCC就是這個(gè)倒頻譜圖的幅度(amplitudes)。一般使用12個(gè)系數(shù),與訊框能量疊加得13維的系數(shù)。
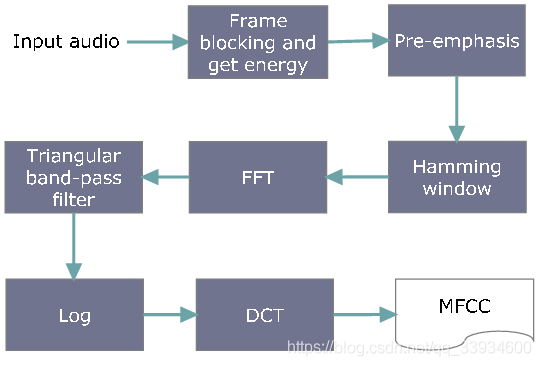
數(shù)據(jù)集
數(shù)據(jù)集來(lái)自Eating Sound Collection,數(shù)據(jù)集中包含20種不同食物的咀嚼聲音,賽題任務(wù)是給這些聲音數(shù)據(jù)建模,準(zhǔn)確分類。
類別包括: aloe, ice-cream, ribs, chocolate, cabbage, candied_fruits, soup, jelly, grapes, pizza, gummies, salmon, wings, burger, pickles, carrots, fries, chips, noodles, drinks
訓(xùn)練集的大小: 750
測(cè)試集的大小: 250
1 下載和解壓數(shù)據(jù)集
!wget http://tianchi-competition.oss-cn-hangzhou.aliyuncs.com/531887/train_sample.zip
!unzip -qq train_sample.zip
!\rm train_sample.zip
!wget http://tianchi-competition.oss-cn-hangzhou.aliyuncs.com/531887/test_a.zip
!unzip -qq test_a.zip
!\rm test_a.zip
2 加載庫(kù)函數(shù)
# 基本庫(kù)
import pandas as pd
import numpy as np
from sklearn.model_selection import train_test_split #劃分?jǐn)?shù)據(jù)集
from sklearn.metrics import classification_report #用于顯示主要分類指標(biāo)的文本報(bào)告
from sklearn.model_selection import GridSearchCV #自動(dòng)調(diào)參
from sklearn.preprocessing import MinMaxScaler #歸一化
加載深度學(xué)習(xí)框架
# 搭建分類模型所需要的庫(kù)
from tensorflow.keras.models import Sequential
from tensorflow.keras.layers import Conv2D, Flatten, Dense, MaxPool2D, Dropout
from tensorflow.keras.utils import to_categorical
from sklearn.ensemble import RandomForestClassifier
from sklearn.svm import SVC #支持向量分類
!pip install librosa --user #加載音頻處理庫(kù)
# 其他庫(kù)
import os
import librosa #音頻處理庫(kù)
import librosa.display
import glob
3 特征提取以及數(shù)據(jù)集的建立
建立類別標(biāo)簽字典
feature = []
label = []
# 建立類別標(biāo)簽,不同類別對(duì)應(yīng)不同的數(shù)字。
label_dict = {'aloe': 0, 'burger': 1, 'cabbage': 2,'candied_fruits':3, 'carrots': 4, 'chips':5,
'chocolate': 6, 'drinks': 7, 'fries': 8, 'grapes': 9, 'gummies': 10, 'ice-cream':11,
'jelly': 12, 'noodles': 13, 'pickles': 14, 'pizza': 15, 'ribs': 16, 'salmon':17,
'soup': 18, 'wings': 19}
label_dict_inv = {v:k for k,v in label_dict.items()}
提取梅爾頻譜特征
from tqdm import tqdm
def extract_features(parent_dir, sub_dirs, max_file=10, file_ext="*.wav"):
c = 0
label, feature = [], []
for sub_dir in sub_dirs:
for fn in tqdm(glob.glob(os.path.join(parent_dir, sub_dir, file_ext))[:max_file]): # 遍歷數(shù)據(jù)集的所有文件
# segment_log_specgrams, segment_labels = [], []
#sound_clip,sr = librosa.load(fn)
#print(fn)
label_name = fn.split('/')[-2]
label.extend([label_dict[label_name]])
X, sample_rate = librosa.load(fn,res_type='kaiser_fast')
mels = np.mean(librosa.feature.melspectrogram(y=X,sr=sample_rate).T,axis=0) # 計(jì)算梅爾頻譜(mel spectrogram),并把它作為特征
feature.extend([mels])
return [feature, label]
# 自己更改目錄
parent_dir = './train_sample/'
save_dir = "./"
folds = sub_dirs = np.array(['aloe','burger','cabbage','candied_fruits',
'carrots','chips','chocolate','drinks','fries',
'grapes','gummies','ice-cream','jelly','noodles','pickles',
'pizza','ribs','salmon','soup','wings'])
# 獲取特征feature以及類別的label
temp = extract_features(parent_dir,sub_dirs,max_file=100)
temp = np.array(temp)
data = temp.transpose()
獲取特征和標(biāo)簽
# 獲取特征
X = np.vstack(data[:, 0])
# 獲取標(biāo)簽
Y = np.array(data[:, 1])
print('X的特征尺寸是:',X.shape)
print('Y的特征尺寸是:',Y.shape)
X的特征尺寸是: (1000, 128)
Y的特征尺寸是: (1000,)
獨(dú)熱編碼
# 在Keras庫(kù)中:to_categorical就是將類別向量轉(zhuǎn)換為二進(jìn)制(只有0和1)的矩陣類型表示
Y = to_categorical(Y)
print(X.shape)
print(Y.shape)
(1000, 128)
(1000, 20)
把數(shù)據(jù)集劃分為訓(xùn)練集和測(cè)試集
X_train, X_test, Y_train, Y_test = train_test_split(X, Y, random_state = 1, stratify=Y)
print('訓(xùn)練集的大小',len(X_train))
print('測(cè)試集的大小',len(X_test))
訓(xùn)練集的大小 750
測(cè)試集的大小 250
X_train = X_train.reshape(-1, 16, 8, 1)
X_test = X_test.reshape(-1, 16, 8, 1)
4 建立模型
搭建CNN網(wǎng)絡(luò)
model = Sequential()
# 輸入的大小
input_dim = (16, 8, 1)
model.add(Conv2D(64, (3, 3), padding = "same", activation = "tanh", input_shape = input_dim))# 卷積層
model.add(MaxPool2D(pool_size=(2, 2)))# 最大池化
model.add(Conv2D(128, (3, 3), padding = "same", activation = "tanh")) #卷積層
model.add(MaxPool2D(pool_size=(2, 2))) # 最大池化層
model.add(Dropout(0.1))
model.add(Flatten()) # 展開(kāi)
model.add(Dense(1024, activation = "tanh"))
model.add(Dense(20, activation = "softmax")) # 輸出層:20個(gè)units輸出20個(gè)類的概率
# 編譯模型,設(shè)置損失函數(shù),優(yōu)化方法以及評(píng)價(jià)標(biāo)準(zhǔn)
model.compile(optimizer = 'adam', loss = 'categorical_crossentropy', metrics = ['accuracy'])
model.summary()
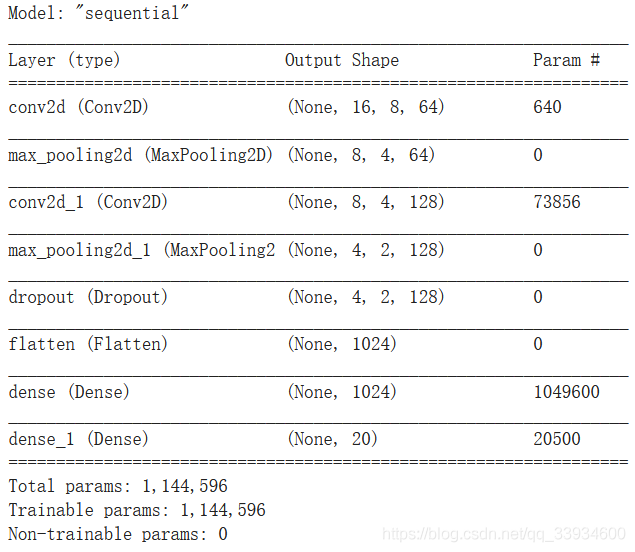
訓(xùn)練模型
# 訓(xùn)練模型
model.fit(X_train, Y_train, epochs = 100, batch_size = 15, validation_data = (X_test, Y_test))
5 預(yù)測(cè)測(cè)試集
def extract_features(test_dir, file_ext="*.wav"):
feature = []
for fn in tqdm(glob.glob(os.path.join(test_dir, file_ext))[:]): # 遍歷數(shù)據(jù)集的所有文件
X, sample_rate = librosa.load(fn,res_type='kaiser_fast')
mels = np.mean(librosa.feature.melspectrogram(y=X,sr=sample_rate).T,axis=0) # 計(jì)算梅爾頻譜(mel spectrogram),并把它作為特征
feature.extend([mels])
return feature
X_test = extract_features('./test_a/')
X_test = np.vstack(X_test)
predictions = model.predict(X_test.reshape(-1, 16, 8, 1))
preds = np.argmax(predictions, axis = 1)
preds = [label_dict_inv[x] for x in preds]
path = glob.glob('./test_a/*.wav')
result = pd.DataFrame({'name':path, 'label': preds})
result['name'] = result['name'].apply(lambda x: x.split('/')[-1])
result.to_csv('submit.csv',index=None)
!ls ./test_a/*.wav | wc -l
!wc -l submit.csv
6 結(jié)果
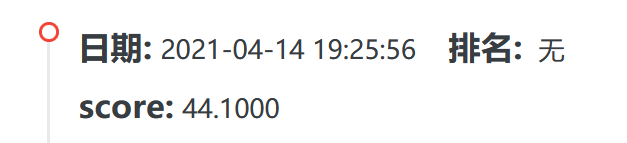
到此這篇關(guān)于基礎(chǔ)語(yǔ)音識(shí)別-食物語(yǔ)音識(shí)別baseline(CNN)的文章就介紹到這了,更多相關(guān)語(yǔ)音識(shí)別的內(nèi)容請(qǐng)搜索腳本之家以前的文章或繼續(xù)瀏覽下面的相關(guān)文章,希望大家以后多多支持腳本之家!
您可能感興趣的文章:- 用Python做個(gè)個(gè)性的動(dòng)畫(huà)掛件讓桌面不單調(diào)
- 自己用python做的一款超炫酷音樂(lè)播放器
- Python做個(gè)自定義動(dòng)態(tài)壁紙還可以放視頻
- 使用python svm實(shí)現(xiàn)直接可用的手寫(xiě)數(shù)字識(shí)別
- 人臉識(shí)別具體案例(李智恩)
- 詳細(xì)過(guò)程帶你用Python做車(chē)牌自動(dòng)識(shí)別系統(tǒng)